Advancing proteomics and machine learning in the clinic: an editorial on “Noninvasive proteomic biomarkers for alcohol-related liver disease”
Alcohol-associated liver disease (ALD) is increasingly prevalent throughout the world (1). It remains the leading cause of liver-related morbidity and mortality worldwide and contributes to approximately 6% of global deaths (2). ALD encompasses a wide range of hepatic pathologies, including steatosis, steatohepatitis, fibrosis, and cirrhosis (3). Given the severe lack of therapeutic interventions available to reverse the progression of ALD, it is currently the leading indication for liver transplantation (4). Advancements within the field are needed to identify diagnostic biomarkers for early detection and to develop therapeutics to ameliorate hepatic dysfunction and pathologies associated with ALD.
The consequence of alcohol toxicity and resulting biochemical perturbations in hepatocytes include disruptions in genetic, proteomic, and metabolic processes, which are largely driven by alcohol metabolism, oxidative stress, and chronic inflammation (5,6). Given the lack of therapeutic options, identifying biological mechanisms and novel targets remains a key focus in the field. Because the liver coordinates whole-body homestasis, hepatic dysfunction and disease progression also influence extrahepatic tissues, in part, via circulating factors in the blood (3). Considering these factors, utilizing high sensitivity approaches to profile proteomic signatures more thoroughly in various compartments of the body are required to address current gaps in knowledge. Importantly, through the novel characterization of clinical biopsies and samples, researchers can discover altered proteomic and metabolomic pathways which will aid in the development of therapies to ameliorate ALD (7,8).
In their article in Nature Medicine, Niu et al. provided an innovative approach profiling paired liver tissue and plasma samples through mass spectrometry (MS)-based proteomics in conjunction with clinical characterization of a large patient cohort of 596 individuals consisting of patients that were alcohol misusers, had early-stage asymptomatic ALD, or were healthy controls (9). In an effort to identify novel diagnostic biomarkers, Niu et al. identified key markers from pathways related to fibrosis, inflammation, and steatosis consistent with other studies (Figure 1) (10-12). Here, they made use of a cutting-edge open-source interactive data exploration tool to identify biomarker panels associated with clinical presentation. Most importantly, the authors developed a machine learning pipeline to deeply examine their plasma proteomics dataset, yielding an exceptional platform for future innovation in classifying diagnostic, prognostic, and therapeutic features in a timely manner related to the clinical presentation of ALD. The robustness of the current study, combined with the identification of novel biomarkers associated with ALD provides an exciting example of the ongoing integration of proteomics analyses within the clinic.
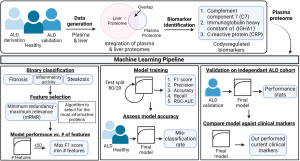
One drawback of OMIC-based clinical analysis is the lack of standardized rigorous methodology for translating high-dimensional and meaningful data that can influence clinical outcomes. While providing targets for future mechanistic work, these approaches are a largely limited to the realm of descriptive research rather than clinically relevant diagnostic/prognostic applications. Additionally, one of the major gaps in ALD research is the lack of predictive biomarkers to direct specific drug targets for fibrosis, inflammation, and steatosis. This supports current knowledge deficits related to early diagnosis, as patients often fail to come to the clinic until they are quite ill and present with advanced disease (e.g., cirrhosis or alcohol-associated hepatitis). Niu et al. leveraged machine learning to bridge these gaps in proteomic and ALD research data analytics. Here, making use of machine learning algorithms to distill a high-dimensional proteomics data set yielded a compact panel of clinically relevant biomarkers and built several ALD-related prediction models. The findings from this study highlight the probability of utilizing these platforms for diagnosis. Importantly, these models demonstrated an ability to outperform the clinical assays that are currently used for diagnosis.
Niu et al. developed a straightforward and highly interpretable workflow to discover a novel biomarker panel for ALD. The plasma proteomics data were processed by filtering for proteins that had valid measurements in at least 60% of samples and were then subject to statistical analysis or machine learning applications. The authors used the resulting dataset to model three separate binary classification targets: significant fibrosis (F0-1 versus F2-4), mild inflammatory activity (I0-1 versus I2-5), and liver steatosis (S0 versus S1-3). Binary classification groupings were determined based on clinical relevance of disease progression and logistic regression classifiers were used to model each outcome. Feature selection was performed upstream of modeling. The goal of feature selection was to choose the smallest set of proteins that would maximize performance. Limiting the number of features used for logistic regression prevents overfitting and improves the predictive ability of the resultant model. To do this, the authors used an implementation of the minimum redundancy-maximum relevance (mRMR) feature selection algorithm and obtained a ranked set of the 50 most important proteins for prediction. Fifty logistic regression models were then built per binary classification target, each using a different number of the top-ranked proteins. The optimal number of proteins was determined for each target by selecting the corresponding model that returned the maximal F1 score. This resulted in three final models (one model per disease target). Nine, six, and twelve features, or proteins, were deemed optimal for the significant fibrosis, advanced inflammation, and steatosis models, respectively. Model performance was evaluated in the discovery cohort using cross-validation and clinical comparators for baseline. The final proteomics models showed improved performance with respect to predicting clinical outcomes as compared to current clinical standards for liver disease identification.
Demonstrating the clinical applicability of these proteomic data within the clinic and robustly identifying ALD biomarkers through a novel machine learning pipeline is a key advancement within the field. Additionally, the researchers generated a blueprint for integrating OMIC data within the clinical setting for liver disease stratification. By displaying that the integration of proteomics into the clinic would improve clinical diagnostics by outperforming current standards for diagnosis these results advance the field toward standardizing integrated proteomics analyses in clinical outcomes. With a disease such as ALD, there is an immediate need for improved, less invasive, rapidly executed, and more sensitive health assessments. This research presents a viable solution to fill this need by integrating proteomic research and clinical application.
The authors addressed several limitations to their study including (I) lack of a universally accepted system for scoring the clinical spectrum of ALD; (II) the potential for blood contamination in hepatic samples; and (III) consideration of the cost-effectiveness of this advanced approach versus the current gold-standard methodology. We would note one additional limitation being a lack of ethnic and racial diversity among patient cohorts, limiting the population these results can be applied to and potentially diminishing the application of their findings to disparate health outcomes. Furthermore, there is often large variability across clinical lab sites regarding performance of proteomic analyses due to sample preparation and instrumentation, resulting in the potential for large differences in quantitative OMIC analyses.
Overall, this recent article provides an exceptional machine learning pipeline and creative bioinformatics approach to reveal novel biomarker panels with diagnostic potential for ALD. Validating their methodology against an independent cohort corroborates the robust and accurate nature of this report. Critically, this clinically relevant analytical workflow is proposed for future studies across myriad hepatic diseases, among others, with the goal of identifying biomarker panels capable of stratifying a range of liver diseases present in the clinic.
Acknowledgments
Funding: This work was funded in part by National Institutes of Health grants NIH R21AA026928, R01DK109964, and AA029218 (K.S.F) and by NIH/NCATS Colorado CTSA Grant Number TL1 TR002533 (C.D.M.). Contents are the authors’ sole responsibility and do not necessarily represent official NIH views.
Footnote
Provenance and Peer Review: This article was commissioned by the editorial office, Hepatobiliary Surgery and Nutrition. The article did not undergo external peer review.
Conflicts of Interest: All authors have completed the ICMJE uniform disclosure form (available at https://hbsn.amegroups.com/article/view/10.21037/hbsn-22-390/coif). The authors have no conflicts of interest to declare.
Ethical Statement: The authors are accountable for all aspects of the work in ensuring that questions related to the accuracy and integrity of any part of the work are appropriately investigated and resolved.
Open Access Statement: This is an Open Access article distributed in accordance with the Creative Commons Attribution-NonCommercial-NoDerivs 4.0 International License (CC BY-NC-ND 4.0), which permits the non-commercial replication and distribution of the article with the strict proviso that no changes or edits are made and the original work is properly cited (including links to both the formal publication through the relevant DOI and the license). See: https://creativecommons.org/licenses/by-nc-nd/4.0/.
References
- Singal AK, Kwo P, Kwong A, et al. Research methodologies to address clinical unmet needs and challenges in alcohol-associated liver disease. Hepatology 2022;75:1026-37. [Crossref] [PubMed]
- World Health Organization. Management of Substance Abuse Team. Global status report on alcohol and health. Geneva, Switzerland: World Health Organization; 2011: xii, 286.
- Poole LG, Dolin CE, Arteel GE. Organ-Organ Crosstalk and Alcoholic Liver Disease. Biomolecules 2017;7:62. [Crossref] [PubMed]
- Szabo G, Thursz M, Shah VH. Therapeutic advances in alcohol-associated hepatitis. J Hepatol 2022;76:1279-90. [Crossref] [PubMed]
- Schnabl B, Arteel GE, Stickel F, et al. Liver specific, systemic and genetic contributors to alcohol-related liver disease progression. Z Gastroenterol 2022;60:36-44. [Crossref] [PubMed]
- Seitz HK, Lieber CS, Stickel F, et al. Alcoholic liver disease: from pathophysiology to therapy. Alcohol Clin Exp Res 2005;29:1276-81. [Crossref] [PubMed]
- Jaurigue MM, Cappell MS. Therapy for alcoholic liver disease. World J Gastroenterol 2014;20:2143-58. [Crossref] [PubMed]
- Singal AK, Shah VH. Current trials and novel therapeutic targets for alcoholic hepatitis. J Hepatol 2019;70:305-13. [Crossref] [PubMed]
- Niu L, Thiele M, Geyer PE, et al. Noninvasive proteomic biomarkers for alcohol-related liver disease. Nat Med 2022;28:1277-87. [Crossref] [PubMed]
- Harris PS, Michel CR, Yun Y, et al. Proteomic analysis of alcohol-associated hepatitis reveals glycoprotein NMB (GPNMB) as a novel hepatic and serum biomarker. Alcohol 2022;99:35-48. [Crossref] [PubMed]
- Massey V, Parrish A, Argemi J, et al. Integrated Multiomics Reveals Glucose Use Reprogramming and Identifies a Novel Hexokinase in Alcoholic Hepatitis. Gastroenterology 2021;160:1725-40.e2. [Crossref] [PubMed]
- Gala KS, Vatsalya V. Emerging Noninvasive Biomarkers, and Medical Management Strategies for Alcoholic Hepatitis: Present Understanding and Scope. Cells 2020;9:524. [Crossref] [PubMed]